In another blog post, I talked about the dangers of over-segmentation. Although I said segmentation is helpful, I didn’t elaborate on how segmentation should be done. Some readers even thought I was disparaging segmentation, which wasn’t my intent.
That post was aimed at helping marketers who have become over-enamoured with the promise of technology alone solving marketing optimization challenges.
Segmentation should indeed be a part of your conversion optimization strategy. Allow me to elaborate today about how we see effective segmentation working.
What is segmentation for conversion optimization?
Though the discussion about segmentation is often about which tools to select, that misses the point. Instead, you should start by thinking about the problem segmentation is attempting to solve.
Here’s the key: segmentation is all about Relevance.
Segmentation is nothing more than a tactic that can help you improve Relevance, one of the six factors in the LIFT Model.
How I Define Segmentation
Segmentation
In the context of conversion optimization, segmentation means putting structures in place to deliver appropriate messages to audiences with distinct needs and expectations.
The important bit in that definition is “distinct needs and expectations.” While there are tools that will target infinitesimally smaller segments based on small data hints and guesses, it can become spurious quickly, if it’s improperly tested.
There may be a potential difference in conversion rate between New York Times readers who research Mazda vehicles in Tennessee on sunny days (if any exist) and USA Today Volkswagen shoppers in Washington when it rains. But, how do you know that to be true? Is it a fact or an assumption?
You should test that!
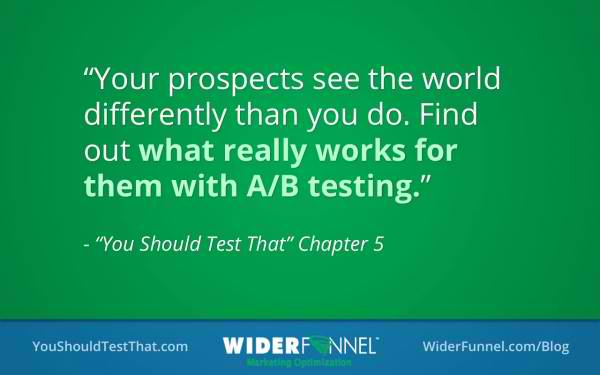
The 8 Steps to Test Segmentation (and anything else too!)
Let’s look at how to create and test the most effective website segmentation in 8 steps.
First, observe
Web Analytics and segmentation tools are awesomely helpful here when they can look at big data, help you identify patterns, and give you fodder for hypothesis-creation. If you can pull in third-party data to gain richer insights into your prospects demographics, psychographics and behaviour, you’ll have even more patterns to observe. That’s the starting point.
Second, hypothesize
Once you’ve identified potential patterns in the data, you need to develop hypotheses about how those patterns could perform with your audience moving forward. Remember that past patterns can often be misleading or simply caused by data clumping. As any good stockbroker will tell you, “Past performance does not guarantee future performance.”
This is where a lot of mistakes are made in segmentation. If you assume patterns you’ve observed are stable without testing against a control group, you’re likely to make a major error.
Get Conversion Optimization Tips from the Experts!
Attend Conversion Conference – 36 sessions focused on the latest strategies and proven tactics by the world’s highest converting websites.
Third, test
A controlled test involves more than just implementing the assumed segments and seeing if they “work.” You need to A/B test your segmentation hypotheses against a control group where segments are not in place.
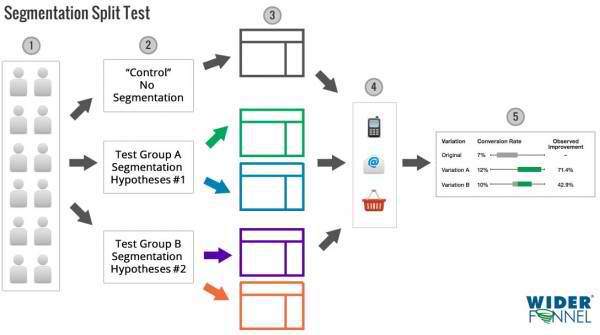
- Select a representative sample of your visitors
- Create alternative segmentation hypotheses
- Select test groups randomly from your visitor sample
- Track conversions based on your most important goals
- Compare performance of each segmentation hypothesis
Fourth, analyze
How did the alternative hypotheses perform? Make sure you analyze the results based on metrics that lead to real business revenue. We recently ran a test where a variation that showed a 60% lift in clicks to the final step in the purchase process showed no different in revenue at all. Make sure you optimize for the right goals.
Fifth, infer
The advanced step in results analysis is to create inferences about the “Why” behind the test results. Why did a certain segment respond differently than another? In science, an inference is made when the cause behind a test result is unknowable or not practical to discover.
In science, an inference is like an educated guess about a cause.
Here’s a helpful presentation from a middle school teacher that explains the difference between scientific observations and inferences.
Thinking about a website example, if you were to find that segmenting landing pages by a visitor’s stated hobby interest, you could make inferences about what those interests mean about the audience segments. You can’t directly measure why that’s the case. To get a direct observation about people’s interest, you’d need to directly survey or hook up every visitor in that test to an fMRI machine. Clearly, neither option would be practical or even possible in a statistically significant A/B test.
A recent WiderFunnel test for a client revealed interesting differences in segmentation conversion rates. We tested a single product landing page for a company with high brand awareness. The test strategy involved various landing page layouts as well as varying amounts of copywriting. The key difference between two of the pages was the form placement.
In one layout, the transaction form was above the fold in the right column. In an alternative variation, there was a button in that spot leading to the full form, which had been moved down the page below longer copywriting content.
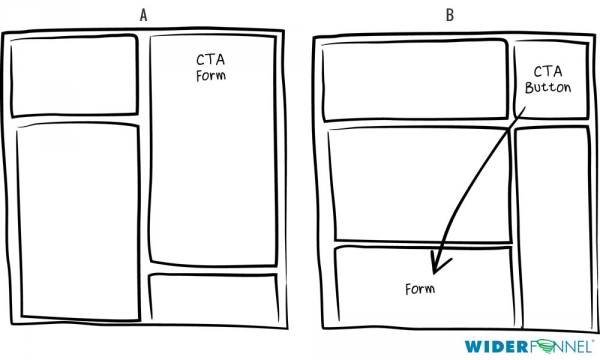
It turned out that a specific traffic source from paid search traffic responded much better to the shorter copy version while the majority of visitors converted at a higher rate on the longer copy version.
Now that we have a data point showing this unique response, we can infer a reason behind that conversion rate difference. Perhaps the paid search target segment needed less convincing. More content for them was just a distraction. The great news is that this segment occurs on many websites, so we have a vast field of opportunities to validate this inference.
To be clear, a single test doesn’t prove this to be true. We’re making an inference that still needs to be validated.
What I’m talking about here is looking for the why behind the what. Don’t just be satisfied with finding a conversion rate lift. Aiming to understand the reason behind the result can lead to greater learning.
Sixth, validate
Here’s another area where mistakes are made. Once you confirm the reason behind a result, you haven’t really learned any substantial principles until it’s validated. Until you’ve found a robust pattern, you know nothing about the reason behind the result.
Thinking about this landing page segmentation example, we can now validate that learning against other landing pages in the same company.
Seventh, theorize
But, we don’t have to stop there. Here’s where it gets exciting.
Looking at a series of similar tests in similar situations allows you to develop a theory about people and their responses. Theories are what lead you to robust scientific marketing learning and insight into how and why people act the way they do online.
Theories are great, but even a theory isn’t very useful until it is predictive.
Eighth, predict and test again
Now that you have a theory developed, you need to put it to the test. A robust theory is one that will predict outcomes.
If you can identify a user behaviour scenario that matches your theory, you can predict the outcomes using your theory. Every successful outcome that your theory predicts strengthens its validity, giving you a powerful strategic tool in your conversion optimization arsenal.
That’s our goal at Widerfunnel, to build the world’s largest database of tested learning and robust marketing theories that will continue to deliver huge continuous website improvement for our clients. Over and over and over.
What do you think?
What’s your experience with web segmentation? What segments are most important in your audience?
This post originally appeared on Business2Community, republished with permission from the author.
About the Author
Chris Goward is author of the book “You Should Test That,” founder of WiderFunnel, and the brain behind the LIFT Model™ and WiderFunnel System™, conversion optimization strategies that consistently lift results for leading companies such as Google, Electronic Arts, ebay, Magento, DMV.org and BuildDirect.com.
Since 2007, Chris has spoken at 150+ events in more than 50 cities around the world. Some of those speaking engagements have included past Conversion Conference events, where he has consistently received top reviews from attendees.